· Research Team · 5 min read
Financial Markets, Unpredictability, and Uncertainty
Why are predicting financial markets nearly impossibe?
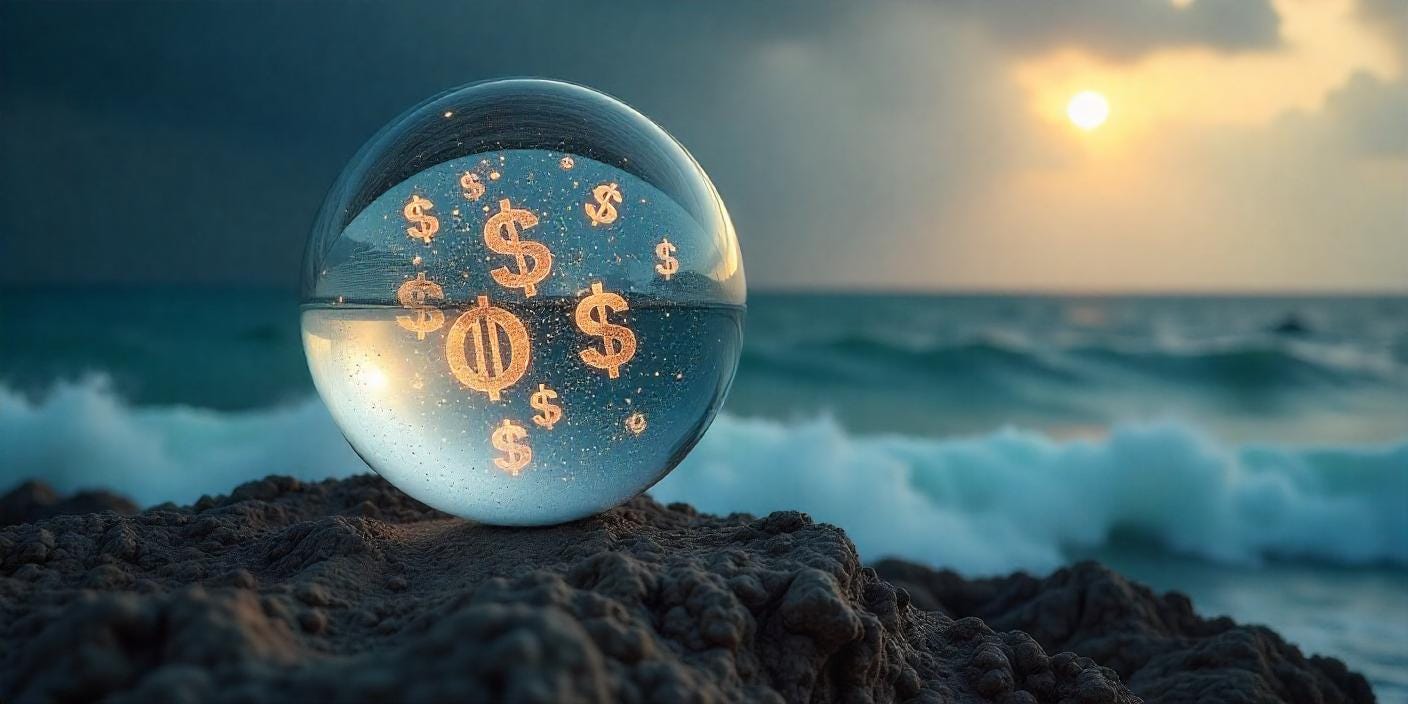
The pursuit of predicting stock market behavior has long tantalized investors, economists, and data scientists alike. Yet, despite advances in technology and quantitative modeling, the markets remain stubbornly resistant to prophecy. This resistance is not a failure of human ingenuity but a reflection of the intrinsic nature of financial systems as dynamic, adaptive, and deeply intertwined with human psychology. Below, we expand on the structural, behavioral, and theoretical forces that render stock markets a domain of profound uncertainty.
The Dual Realities of Chaos: From Sunspots to Self-Sabotage
Chaos theory delineates systems into two categories. Level-one chaos systems, such as solar activity or planetary motion, operate independently of observation. Their trajectories remain unaffected by human analysis. Financial markets, however, reside firmly in the realm of level-two chaos, where the act of prediction alters the predicted outcome.
Consider a hypothetical scenario: a widely publicized model forecasts a 20% decline in the S&P 500 within a month. Institutional investors, fearing losses, may preemptively sell assets, triggering the very crash the model sought to anticipate. Not only is there a dataset shift but also there is a model-(structure)-shift in time series. This phenomenon termed the “prophet’s dilemma” or “self-defeating prediction,” underscores a critical paradox: the more influential a prediction, the less reliable it becomes. Markets are not passive datasets but reactive ecosystems, shaped by the collective actions of those who study them.
Non-Stationarity: The Shifting Sands of Financial Data
Financial markets are inherently non-stationary—their statistical properties, such as volatility and correlation structures, evolve over time. A trading strategy that thrived in the 1990s may falter today, not due to flawed logic but because the market’s “rules” have changed. Factors such as algorithmic trading, geopolitical shocks, and regulatory shifts continuously rewrite the market’s playbook.
For instance, the rise of retail trading platforms and meme-stock mania in 2021 upended traditional liquidity models. Similarly, the 2008 global financial crisis revealed that historical correlations between asset classes can dissolve overnight. Non-stationarity ensures that past patterns are unreliable guides to future behavior, leaving even the most sophisticated models vulnerable to structural breaks.
The Limits of Financial Oracles: When Models Meet Madness
Quantitative finance has produced remarkable tools—stochastic calculus, machine learning, and risk-neutral pricing—yet these frameworks struggle to encapsulate the human elements driving markets. Financial models often assume rational actors and efficient information dissemination. Reality, however, is messier.
Behavioral biases—herding, overconfidence, and panic—routinely defy mathematical logic. The 1987 Black Monday crash, where markets plunged 22% in a single day, occurred without clear macroeconomic triggers. Decades later, the GameStop saga demonstrated how collective sentiment, amplified by social media, could decouple stock prices from fundamental value. Models reliant on historical data cannot account for such paradigm shifts, as they exist outside the bounds of quantifiable precedent.
Adaptive Strategies: Navigating Uncertainty with Discipline
If prediction is futile, what remains for investors? The answer lies in robust adaptation. Successful market participation requires acknowledging uncertainty rather than resisting it. Strategies such as diversification, dynamic asset allocation, and scenario planning prioritize resilience over prescience.
Warren Buffett’s enduring adage—“Be fearful when others are greedy, and greedy when others are fearful”—encapsulates this philosophy. By focusing on the margin of safety, long-term horizons, and businesses with durable competitive advantages, investors mitigate the risks of short-term volatility. Similarly, trend-following algorithms, which adapt to shifting market regimes, exemplify how systematic approaches can thrive without relying on static predictions.
The Role of Behavioral Economics: Mapping the Human Quotient
Modern finance increasingly recognizes that markets are not merely numerical constructs but psychological arenas. Behavioral economics, pioneered by figures like Daniel Kahneman and Richard Thaler, illuminates how cognitive biases distort decision-making. Anchoring, recency bias, and loss aversion explain why investors often buy high (during euphoria) and sell low (amid panic).
These biases are not anomalies but systemic features of market behavior. For example, the dot-com bubble of the late 1990s saw investors irrationally extrapolate perpetual growth from nascent internet firms. When the bubble burst, it revealed the peril of conflating optimism with analysis. Understanding these tendencies does not eliminate uncertainty, but it equips participants to recognize—and occasionally exploit—the gaps between perception and reality.
Conclusion: Embracing Uncertainty as the Only Certainty
The stock market’s defiance of prediction is not a flaw but a feature of its design. It is a complex adaptive system, shaped by millions of actors, evolving technologies, and unforeseeable global events. To demand predictability from such a system is to misunderstand its essence.
Investors would do well to adopt a mindset of humility and preparedness. This means diversifying across uncorrelated assets, stress-testing portfolios against extreme scenarios, and maintaining liquidity to capitalize on dislocations. As the mathematician Benoît Mandelbrot observed, markets are “wildly random”—a reality that rewards patience, discipline, and respect for the unknown.
In the end, the greatest edge an investor can possess is not a crystal ball but the wisdom to navigate fog.
Data-Driven Decision Making is a reader-supported publication. To receive new posts and support my work, consider becoming a free or paid subscriber.